day04_Flink advanced API
Today's goal
- Flink's four cornerstones
- Flink Window operation
- Flink Time - Time
- Flink Watermark watermark mechanism
- State management of Flink - keyed state and operator state
Flink's four cornerstones
- Checkpoint - checkpoint, distributed consistency, data loss resolution, fault recovery, data storage is the global state, persistent in HDFS distributed file system
- State - state, divided into Managed state and raw state; From the perspective of data structure, ValueState, ListState, MapState and BroadcastState
- Time - time, EventTime, event time, Ingestion time, Process processing time
- Window - window, time window and count window, TimeWindow, countwindow, sessionwindow
Window operation
- Why do I need Window - Window The data is dynamic and unbounded, which requires the window to delimit the scope and convert the unbounded data into bounded and static data for calculation.
Window classification
- Time - sort by time
- Window level of time, day, hour, minute
- Use more scrolling windows - tumbling window s and sliding windows - sliding windows
- Scroll window. Window time is the same as sliding time
- Sliding window, the sliding time is less than the window time;
- Session window - session windows
- Count - count for classification
- Scroll count window
- Sliding count window
How to use
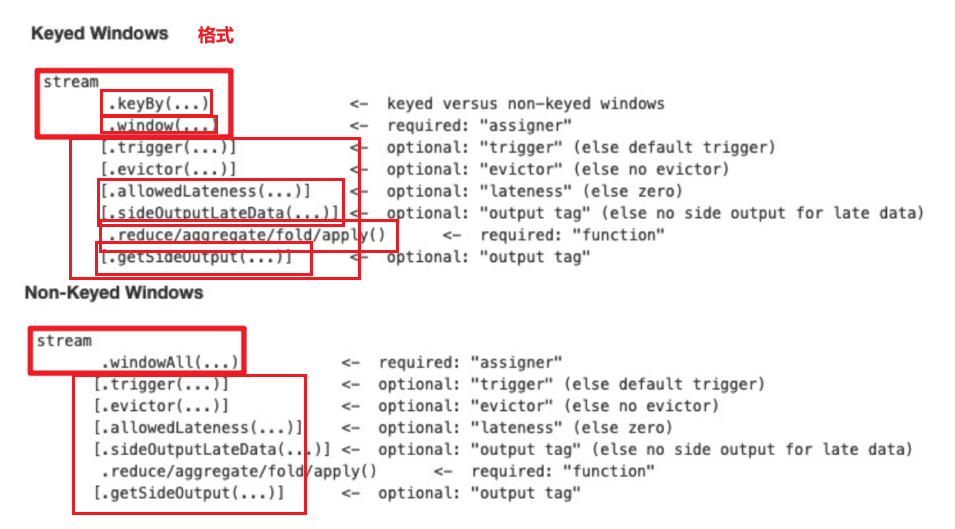
windows case
Time window requirements
- Count the number of vehicles passing traffic lights at each intersection in the last 5 seconds every 5 seconds - time-based scrolling window
- Count the number of vehicles passing traffic lights at each intersection in the last 10 seconds every 5 seconds - time-based sliding window
package cn.itcast.flink.basestone; import lombok.AllArgsConstructor; import lombok.Data; import lombok.NoArgsConstructor; import org.apache.flink.api.common.functions.MapFunction; import org.apache.flink.streaming.api.datastream.DataStream; import org.apache.flink.streaming.api.datastream.DataStreamSource; import org.apache.flink.streaming.api.datastream.SingleOutputStreamOperator; import org.apache.flink.streaming.api.environment.StreamExecutionEnvironment; import org.apache.flink.streaming.api.windowing.assigners.SlidingProcessingTimeWindows; import org.apache.flink.streaming.api.windowing.assigners.TumblingProcessingTimeWindows; import org.apache.flink.streaming.api.windowing.time.Time; /** * Author itcast * Date 2021/6/18 15:00 * Development steps * 1. Convert string 9,3 to CartInfo * 2. Use the scroll window to slide the window * 3. Grouping and aggregation * 4. Printout * 5. execution environment */ public class WindowDemo01 { public static void main(String[] args) throws Exception { //1.env create flow execution environment StreamExecutionEnvironment env = StreamExecutionEnvironment.getExecutionEnvironment(); env.setParallelism(1); //2. Read socket data source DataStreamSource<String> source = env.socketTextStream("192.168.88.161", 9999); //3. Convert 9,3 to CartInfo(9,3) DataStream<CartInfo> mapDS = source.map(new MapFunction<String, CartInfo>() { @Override public CartInfo map(String value) throws Exception { String[] kv = value.split(","); return new CartInfo(kv[0], Integer.parseInt(kv[1])); } }); //4. Group according to sensorId and divide the scrolling window into 5 seconds, and sum on the window // Tumbling processing timewindows //Demand 1: count the number of vehicles passing traffic lights at each intersection / signal in the last 5 seconds every 5 seconds SingleOutputStreamOperator<CartInfo> result1 = mapDS.keyBy(t -> t.sensorId) .window(TumblingProcessingTimeWindows.of(Time.seconds(5))) .sum("count"); //Demand 2: count the number of vehicles passing traffic lights at each intersection / signal in the last 10 seconds every 5 seconds SingleOutputStreamOperator<CartInfo> result2 = mapDS.keyBy(t -> t.sensorId) .window(SlidingProcessingTimeWindows.of(Time.seconds(10),Time.seconds(5))) .sum("count"); //5. Printout //result1.print(); result2.print(); //6.execute env.execute(); } @Data @AllArgsConstructor @NoArgsConstructor public static class CartInfo { private String sensorId;//Signal lamp id private Integer count;//Number of vehicles passing the signal lamp } }
Counting window requirements
- Demand 1: count the number of cars passing through each intersection in the last 5 messages. Count every 5 times the same key appears - a scrolling window based on the number
- Demand 2: count the number of cars passing through each intersection in the last five messages. The same key will be counted every three times - a sliding window based on the number
package cn.itcast.flink.basestone; import lombok.AllArgsConstructor; import lombok.Data; import lombok.NoArgsConstructor; import org.apache.flink.api.common.functions.MapFunction; import org.apache.flink.streaming.api.datastream.DataStream; import org.apache.flink.streaming.api.datastream.DataStreamSource; import org.apache.flink.streaming.api.datastream.SingleOutputStreamOperator; import org.apache.flink.streaming.api.environment.StreamExecutionEnvironment; /** * Author itcast * Date 2021/6/18 15:46 * Desc TODO */ public class CountWindowDemo01 { public static void main(String[] args) throws Exception { //1.env create flow execution environment StreamExecutionEnvironment env = StreamExecutionEnvironment.getExecutionEnvironment(); env.setParallelism(1); //2. Read socket data source DataStreamSource<String> source = env.socketTextStream("192.168.88.161", 9999); //3. Convert 9,3 to CartInfo(9,3) DataStream<WindowDemo01.CartInfo> mapDS = source.map(new MapFunction<String, WindowDemo01.CartInfo>() { @Override public WindowDemo01.CartInfo map(String value) throws Exception { String[] kv = value.split(","); return new WindowDemo01.CartInfo(kv[0], Integer.parseInt(kv[1])); } }); // *Demand 1: count the number of cars passing through each intersection in the last five messages. Count every five times the same key appears -- a rolling window based on the number // //countWindow(long size, long slide) SingleOutputStreamOperator<WindowDemo01.CartInfo> result1 = mapDS.keyBy(t -> t.getSensorId()) .countWindow(5) .sum("count"); // *Demand 2: count the number of cars passing through each intersection in the last five messages. The same key is counted every three times -- a sliding window based on the number SingleOutputStreamOperator<WindowDemo01.CartInfo> result2 = mapDS.keyBy(t -> t.getSensorId()) .countWindow(5, 3) .sum("count"); //Printout //result1.print(); result2.print(); //execution environment env.execute(); } @Data @AllArgsConstructor @NoArgsConstructor public static class CartInfo { private String sensorId;//Signal lamp id private Integer count;//Number of vehicles passing the signal lamp } } package cn.itcast.flink.basestone; import lombok.AllArgsConstructor; import lombok.Data; import lombok.NoArgsConstructor; import org.apache.flink.api.common.functions.MapFunction; import org.apache.flink.streaming.api.datastream.DataStream; import org.apache.flink.streaming.api.datastream.DataStreamSource; import org.apache.flink.streaming.api.datastream.SingleOutputStreamOperator; import org.apache.flink.streaming.api.environment.StreamExecutionEnvironment; /** * Author itcast * Date 2021/6/18 15:46 * Desc TODO */ public class CountWindowDemo01 { public static void main(String[] args) throws Exception { //1.env create flow execution environment StreamExecutionEnvironment env = StreamExecutionEnvironment.getExecutionEnvironment(); env.setParallelism(1); //2. Read socket data source DataStreamSource<String> source = env.socketTextStream("192.168.88.161", 9999); //3. Convert 9,3 to CartInfo(9,3) DataStream<WindowDemo01.CartInfo> mapDS = source.map(new MapFunction<String, WindowDemo01.CartInfo>() { @Override public WindowDemo01.CartInfo map(String value) throws Exception { String[] kv = value.split(","); return new WindowDemo01.CartInfo(kv[0], Integer.parseInt(kv[1])); } }); // *Demand 1: count the number of cars passing through each intersection in the last five messages. Count every five times the same key appears -- a rolling window based on the number // //countWindow(long size, long slide) SingleOutputStreamOperator<WindowDemo01.CartInfo> result1 = mapDS.keyBy(t -> t.getSensorId()) .countWindow(5) .sum("count"); // *Demand 2: count the number of cars passing through each intersection in the last five messages. The same key is counted every three times -- a sliding window based on the number SingleOutputStreamOperator<WindowDemo01.CartInfo> result2 = mapDS.keyBy(t -> t.getSensorId()) .countWindow(5, 3) .sum("count"); //Printout //result1.print(); result2.print(); //execution environment env.execute(); } @Data @AllArgsConstructor @NoArgsConstructor public static class CartInfo { private String sensorId;//Signal lamp id private Integer count;//Number of vehicles passing the signal lamp } }
Flink - Time and watermark
Time - time
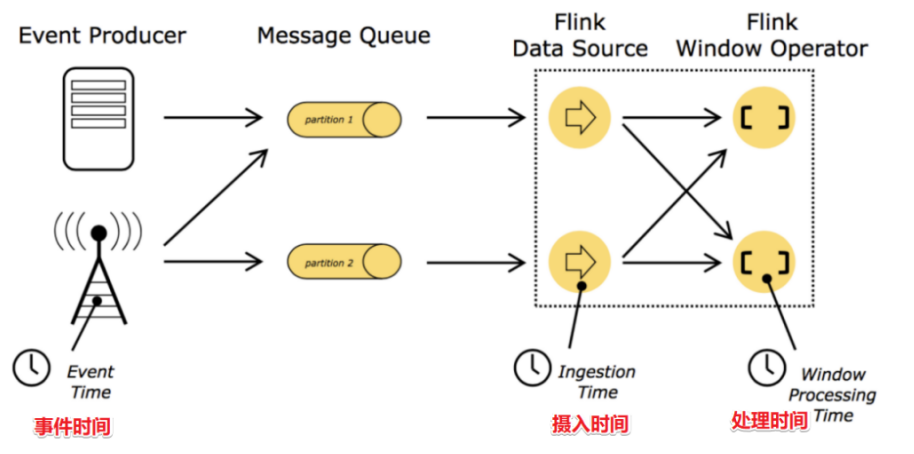
Watermark mechanism - watermark
- It mainly solves the problem of data delay
- Watermark (timestamp) = event time - maximum allowable delay time
- Window trigger condition Watermark time > = the end time of the window triggers the calculation
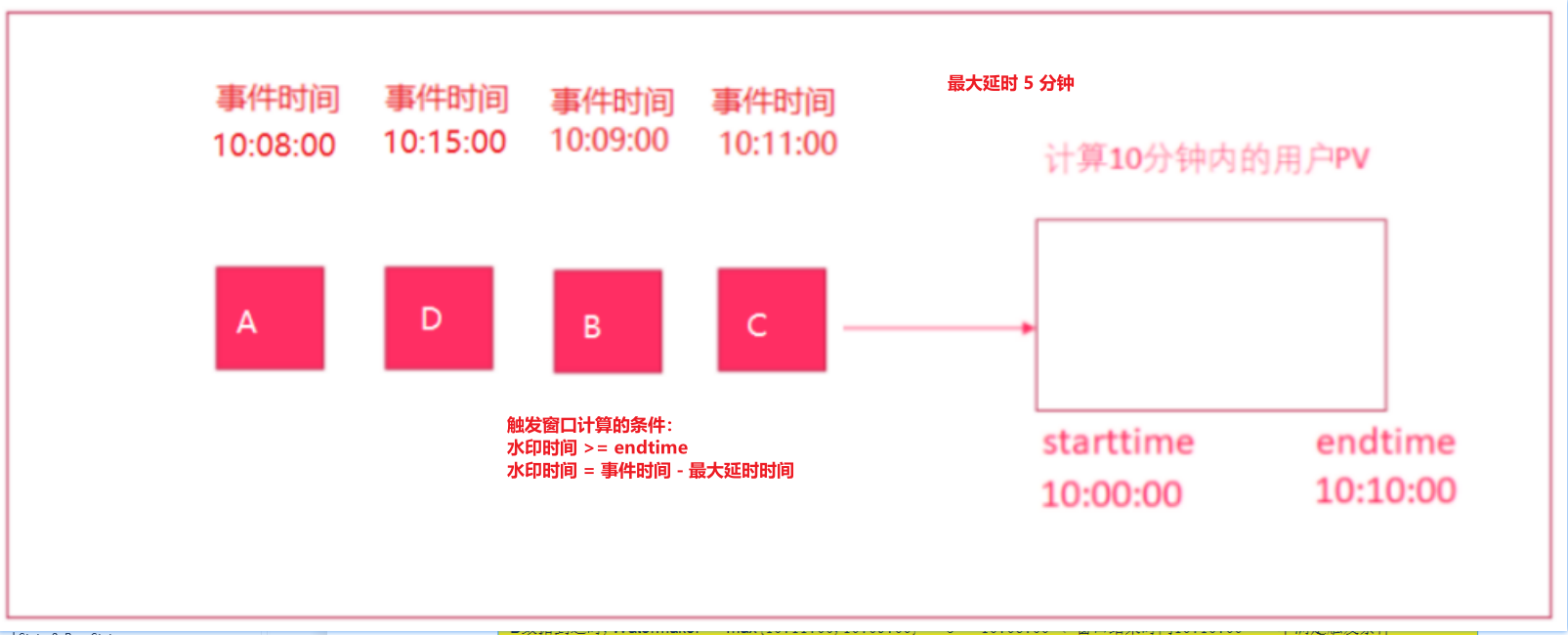
demand
There is order data in the format of: (order ID, user ID, timestamp / event time, order amount)
It is required to calculate the total order amount of each user within 5 seconds every 5s
Watermark is added to solve the problems of data delay and data disorder (up to 3 seconds).
package cn.itcast.flink.basestone; import lombok.AllArgsConstructor; import lombok.Data; import lombok.NoArgsConstructor; import org.apache.flink.api.common.eventtime.WatermarkStrategy; import org.apache.flink.streaming.api.datastream.DataStream; import org.apache.flink.streaming.api.datastream.DataStreamSource; import org.apache.flink.streaming.api.environment.StreamExecutionEnvironment; import org.apache.flink.streaming.api.functions.source.SourceFunction; import org.apache.flink.streaming.api.windowing.assigners.TumblingEventTimeWindows; import org.apache.flink.streaming.api.windowing.time.Time; import java.time.Duration; import java.util.Random; import java.util.UUID; /** * Author itcast * Date 2021/6/18 16:54 * Desc TODO */ public class WatermarkDemo01 { public static void main(String[] args) throws Exception { //1.env StreamExecutionEnvironment env = StreamExecutionEnvironment.getExecutionEnvironment(); //Set the property ProcessingTime. The new version sets EventTime by default //env.setStreamTimeCharacteristic(TimeCharacteristic.EventTime); //2.Source creates an Order class orderId:String userId:Integer money:Integer eventTime:Long DataStreamSource<Order> source = env.addSource(new SourceFunction<Order>() { boolean flag = true; Random rm = new Random(); @Override public void run(SourceContext<Order> ctx) throws Exception { while (flag) { ctx.collect(new Order( UUID.randomUUID().toString(), rm.nextInt(3), rm.nextInt(101), //Simulated generation of Order data event time = current time - 5 seconds random * 1000 System.currentTimeMillis() - rm.nextInt(5) * 1000 )); Thread.sleep(1000); } } @Override public void cancel() { flag = false; } }); //3.Transformation //-Tell Flink to calculate based on the event time! //env.setStreamTimeCharacteristic(TimeCharacteristic.EventTime);// The new version defaults to eventtime DataStream<Order> result = source.assignTimestampsAndWatermarks( WatermarkStrategy.<Order>forBoundedOutOfOrderness(Duration.ofSeconds(3)) .withTimestampAssigner((element, recordTimestamp) -> element.eventTime) ) //-The Watermark mechanism is allocated with a maximum delay of 3 seconds to tell which column in the Flink data is the event time, because Watermark = the current maximum event time - the maximum allowable delay time or out of order time //When the code comes here, Watermark has been added! Next, you can calculate the window //It is required to calculate the total order amount of each user within 5 seconds (time-based scrolling window) every 5s .keyBy(t -> t.userId) .window(TumblingEventTimeWindows.of(Time.seconds(5))) .sum("money"); //4.Sink result.print(); //5.execute env.execute(); } //Create order class @Data @AllArgsConstructor @NoArgsConstructor public static class Order{ private String orderId; private Integer userId; private Integer money; private Long eventTime; } }
- Implementation of watermark mechanism by user-defined rewriting interface
import lombok.AllArgsConstructor; import lombok.Data; import lombok.NoArgsConstructor; import org.apache.flink.api.common.eventtime.WatermarkStrategy; import org.apache.flink.streaming.api.datastream.DataStream; import org.apache.flink.streaming.api.datastream.DataStreamSource; import org.apache.flink.streaming.api.environment.StreamExecutionEnvironment; import org.apache.flink.streaming.api.functions.source.SourceFunction; import org.apache.flink.streaming.api.windowing.assigners.TumblingEventTimeWindows; import org.apache.flink.streaming.api.windowing.time.Time; import java.time.Duration; import java.util.Random; import java.util.UUID; /** * Author itcast * Date 2021/6/18 16:54 * Desc TODO */ public class WatermarkDemo01 { public static void main(String[] args) throws Exception { //1.env StreamExecutionEnvironment env = StreamExecutionEnvironment.getExecutionEnvironment(); //Set the property ProcessingTime. The new version sets EventTime by default //env.setStreamTimeCharacteristic(TimeCharacteristic.EventTime); //2.Source creates an Order class orderId:String userId:Integer money:Integer eventTime:Long DataStreamSource<Order> source = env.addSource(new SourceFunction<Order>() { boolean flag = true; Random rm = new Random(); @Override public void run(SourceContext<Order> ctx) throws Exception { while (flag) { ctx.collect(new Order( UUID.randomUUID().toString(), rm.nextInt(3), rm.nextInt(101), //Simulated generation of Order data event time = current time - 5 seconds random * 1000 System.currentTimeMillis() - rm.nextInt(5) * 1000 )); Thread.sleep(1000); } } @Override public void cancel() { flag = false; } }); //3.Transformation //-Tell Flink to calculate based on the event time! //env.setStreamTimeCharacteristic(TimeCharacteristic.EventTime);// The new version defaults to eventtime DataStream<Order> result = source.assignTimestampsAndWatermarks( WatermarkStrategy.<Order>forBoundedOutOfOrderness(Duration.ofSeconds(3)) .withTimestampAssigner((element, recordTimestamp) -> element.eventTime) ) //-The Watermark mechanism is allocated with a maximum delay of 3 seconds to tell which column in the Flink data is the event time, because Watermark = the current maximum event time - the maximum allowable delay time or out of order time //When the code comes here, Watermark has been added! Next, you can calculate the window //It is required to calculate the total order amount of each user within 5 seconds (time-based scrolling window) every 5s .keyBy(t -> t.userId) .window(TumblingEventTimeWindows.of(Time.seconds(5))) .sum("money"); //4.Sink result.print(); //5.execute env.execute(); } //Create order class @Data @AllArgsConstructor @NoArgsConstructor public static class Order{ private String orderId; private Integer userId; private Integer money; private Long eventTime; } }
Flink status management
- State is the intermediate result based on key or operator
- Flink state is divided into two types: Managed state - Managed state and Raw state - original state
- Managed state s are divided into two types:
- keyed state is based on the state on the key Supported data structures valueState listState mapState broadcastState
- operator state is based on the state of the operation Byte array, ListState
Flink keyed state case
Flink operator state case
IndexOfThisSubtask(); System.out.println("index: "+idx+" offset:"+offset); Thread.sleep(1000); if(offset % 5 ==0){ System.out.println("there is an error in the current program...); throw new Exception("program BUG...); } } } //Override the cancel method @Override public void cancel() { flag = false; }
//Override the snapshotState method, clear the offsetState, and add the latest offset @Override public void snapshotState(FunctionSnapshotContext context) throws Exception { offsetState.clear(); offsetState.add(offset); } }
}